Today's Selfie Is Tomorrow's Biometric Profile
Essay commissioned by Hartware MedienKunstVerein for the House of Mirrors exhibition. Available in book or PDF format.
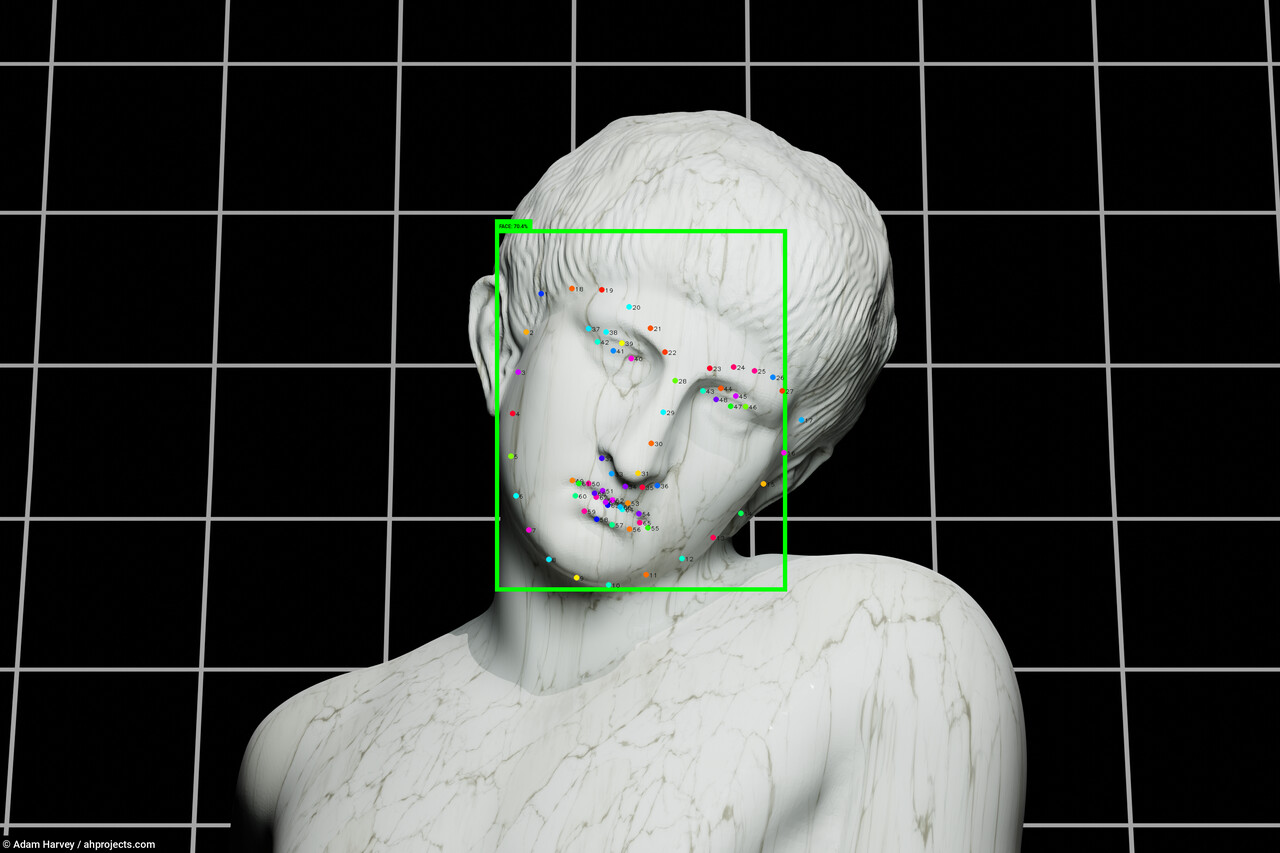
In Greek mythology Narcissus became transfixed and paralyzed by the beauty of his own reflection, ultimately leading to his ruin. Today a similar tragedy is occurring in the reflecting pool of social media where the allure of selfies has contributed to the rise of biometric surveillance technologies. Artwork by Adam Harvey 2022 CC-BY-NC-SA (based on a 3D scan of “Ephebe Narcissus” by Scan The World).
The production of commodities creates, and is the one and universal cause that creates a market for the commodities produced.
— James Mill, Commerce Defended (1808)
In 2021 the IRS (U.S. Internal Revenue Service) announced they would begin requiring “selfies” for face recognition to access tax documents on their website. Their demand was strongly rejected by civil rights groups1, technology activists2, and even politicians from both parties.3 In response, the IRS announced in February 2022 they “will transition away from using a third-party service for facial recognition” and instead “bring online an additional authentication process that does not involve facial recognition.”4
It is the latest example of a precarious global trend towards using selfie-style biometric authentication. Amazon, Google, Intel, MasterCard, and countless financial startups have already launched similar programs using “pay by selfie” technology that utilizes frontal-facing smartphone cameras to authenticate financial transactions. The habituated, comfortable, and expressive action of taking self-portraits is now a high-security interaction. Many airports and border control checkpoints around the world are also using similar selfie-styled face recognition kiosks to screen for potential terrorists and expedite passenger boarding verification.
In the U.S., airlines claim to delete these photos, but there are different retention rules for different nationalities, and exceptions for suspicious persons. Whether or not a traveler’s biometrics are actually deleted remains uncertain and unknowable. The airlines do not actually provide the face recognition technology, only the camera interface. The face recognition algorithms and database of known subjects are operated by the Department for Homeland Security (DHS), Customs and Border Protection, or similar agencies. In order to evaluate the performance of this technology, faces may be retained for quality assurance and to improve the product.
For example, NIST (National Institute of Standards and Technology) compiled a dataset with 1.6M face images from border checkpoints called Visa-Border. The faces were collected to benchmark commercial face recognition technology providers and determine the most accurate algorithms. The dataset enables commercial face recognition technology vendors to evaluate, understand, and improve their algorithms. Benchmark data plays an important role as a diagnostic tool in advancing computer vision. Companies use NIST evaluations to measure progress and understand technical shortcomings. Top ranking companies can also use their scores in advertisements. For example, in 2020 CyberLink boasted that their FaceMe® technology achieves a True Acceptance Rate (TAR) of 98.11% on a database of 1.6 million travelers in the NIST Visa-Border dataset. More recently in October 2021, the controversial face recognition company Clearview AI used their NIST scores for a press release, which was then picked up by media organizations providing free advertising.5 In this way, the non-consensual faces used in the NIST Visa-Border dataset create tangible value for commercial face recognition vendors.
Prior to benchmarking, face recognition algorithms must first be trained on a separate dataset of faces from a different source. The training dataset is typically around 5-10 times larger than than testing datasets. There is no formal metric for how many faces are needed to train, but several million faces with diverse pose, age, gender, skin tone, expression, and image quality are commonly used, with more images typically leading to higher performance. Since higher performance face recognition algorithms are more marketable, companies have an economic incentive to collect as many faces as possible.
To obtain large quantities of facial training data developers often rely on the Internet as a virtually unlimited source of face data. Among the most common sources cited in face recognition research papers and public face recognition datasets are search results from Google, celebrity photos from IMDB.com, selfies from Instagram, YouTube videos, and Creative Commons licensed images from Flickr. Images obtained online without the consent of the subject are referred to by researchers as “media in the wild,” implying that the images originate from real world scenarios. But the Internet does not accurately portray reality; it is a simulation full of bias and commercial eccentricities. Most face datasets suffer from being overwhelmingly white and full of celebrities. This reflects social inequalities embedded in mainstream media (#OscarsSoWhite) and social media platforms which can skew heavily towards affluent tech users with lighter skin tones. When media biases are hard-coded into face recognition training datasets, they trickle down into dangerously incompetent or racist face recognition systems. Numerous articles6 and studies have pointed out the dangers caused by biometric systems when they are built using unrepresentative datasets. For instance, an ACLU study from 2018 found that Amazon’s face recognition incorrectly identified 28 members of the U.S. Congress as criminals in a mugshot database, of which a disproportionate number had darker skin tones.7
The engineering solution to racially incompetent face recognition systems is to add more diverse training data. According to AI expert Kai-Fu Lee “[t]he more data the better the AI works, more brilliantly than how the researcher is working on the problem.”8 Misguided by good intentions, researchers from IBM in 2019 aimed to solve bias in face recognition by creating a more racially diverse dataset, called IBM Diversity in Faces (DiF), with over 1 million faces. They hoped that by providing this data it would help solve representational problems across the face recognition industry. However, the dataset authors failed to understand that non-consensual analysis and distribution of biometric data creates a different set of problems; it violates biometric information protection laws. An ongoing class-action lawsuit against IBM is seeking damages of $1,000 for each negligent violation and $5,000 for each intentional violation of BIPA (Illinois’ Biometric Information Privacy Act) per use, possibly amounting to hundreds of millions in fines.9
The IBM DiF dataset is one among dozens, likely hundreds, of face recognition datasets created “in the wild” by taking faces without consent. During the last several years, research for my Exposing.ai project has shown that tens of millions of faces have been scraped from the Internet and used for training, testing, and enhancing face recognition and other biometric analysis technologies. One of the largest datasets, called MegaFace, used over 4.7M faces taken from Creative Commons licensed Flickr images. Even before #selfies were mainstream, user-generated photo tags in MegaFace show that permutations of #self and #portrait tags were already a common theme in training data, comprising at least 51,230 images.10
Training datasets are highly guarded secrets in the face recognition industry. Companies are reluctant to disclose their data sources out of fear it could cause legal issues. For example, in 2021 an NBC investigation11 revealed that Everalbum, Inc. (now Paravision AI) was charged by the Federal Trade Commission for using millions of users’ faces without consent to build face recognition technologies. The FTC forced Everalbum to delete all biometric data and any face recognition model trained using it.12 Coincidentally, Everalbum’s AI group was listed as a user of the MegaFace dataset of non-consensual Flickr images. Ever AI (now Paravision) also participated in the NIST Visa-Border that uses 1.6M apparently non-consensual face images from border crossings in U.S. It seems that no matter where your selfies are appearing these days, companies and government agencies are eager to use it as biometric data.
To those working in the biometrics industry the word “selfie” is basically shorthand for a biometric profile. The popular biometric industry website, BiometricUpdate.com, yields over 100 pages of results for “selfie” related biometric news dating back to 2014. What was once viewed as form of personal expression on the Internet has been operationalized into security systems. This creates new privacy risks because selfies also contain expressive data and “sociomaterial”13, or performed social identity signals.
To better understand the performative identity component of selfies, a 2017 research study funded by U.S. Army Research Office (ARO) and Defense Advanced Research Projects Agency (DARPA) collected 2.5M images from Instagram that used the hashtag #selfie. The researchers used Erving Goffman’s concept of a performed “front” from his book “The Presentation of Self in Everyday Life” (1959) as a starting point to reconsider how this is played out on social media. Goffman considered “front” as the “expressive equipment” used during the performance of identity. The researchers use Goffman’s idea of a “front” to unerstand how identity is performed on Instagram using custom lighting effects, cropping styles, GPS coordinates, memetic expressions (e.g. duck face), and other forms of socio-technical “expressive equipment” as new types of performed identity data.13
Thinking of selfies in this way, as having an additional layer of performed data, helps to explain misleading pseudo-scientific research projects that claim to use face recognition to detect sexuality14 or political orientation14. In Michal Kosinski’s controversial 2021 research paper “Facial recognition technology can expose political orientation from naturalistic facial images” the data can be interpreted in multiple ways. Kosinski claimed that face recognition can be used to predict political orientation with 73% accuracy, but his data also shows that facial expression attributes (anger, happiness, sadness) and head pose (roll, yaw, pitch) were 80% as effective as the face recognition data. But since face recognition can often produce matches based on head pose and expression (i.e. smiling faces are more likely to match smiling faces and profile views are more like to match profile views) it is more likely that he conflated face recognition with face attribute analysis, and merely decoded the performed front-matter, not the underlying intrinsic facial structure.
Assuming that faces can provide absolute and stable identity data is dubious. Faces, unlike fingerprints, change over time and accrue unique information throughout life. Faces comprise solid data (skull), rigid (cartilage), semi-rigid (expression), soft (facial hair), chronological (wrinkles), and front-matter (performed social signals) biometric data. The multitude of stable, unstable, static, dynamic, and performed identity data types that can supposedly be detected using computer vision make faces uniquely vulnerable to misidentification and misclassification. Numerous research papers claim to be able to detect amusement, age, anger, attentiveness, attractive, autism, awe, beauty, body mass index, boredom, calmness, concentration, confidence, criminality, and depression to name only a few.
One of the driving factors behind the growth of facial analysis technologies and pseudo-scientific research is the surplus of face data available online. By analyzing publicly available datasets, which are the tip of the iceberg because most are private, it becomes clear that as more face images are posted online, more face datasets begin to appear. For example, the UCF Selfie Dataset scraped 46,836 selfies from Instagram to build facial attribute analysis algorithm to estimate age, gender, race, face shape, and face gesture. And, to improve face recognition performance, Microsoft researchers scraped and distributed 10 million face images of 100,000 celebrities in the MS-Celeb dataset, IBM downloaded 1 million faces from Flickr for their DiF dataset, and the University of Washington took 4.7M face images from Flickr for the MegaFace dataset. Coincidentally, MegaFace was used by the CEO of Clearview AI, who now competes in the NIST Visa-Border face recognition challenge to provide biometric technology to government agencies like the Department of Homeland Security. In other words, it is possible your face is or will eventually be used during all stages of face recognition development: training, testing, and deployment.
If supply can be said to create demand, then the surplus of selfies has helped deliver the age of facial surveillance. Today’s selfies are not only tomorrow’s biometric profiles, they are also the growth drivers of future technologies. Selfies beget biometrics. To pay by selfie is also to invest in facial surveillance futures.
© Adam Harvey 2022. All Rights Reserved.
This essay was commissioned by Hartware MedienKunstVerein for the exhibition House of Mirrors: Artificial Intelligence as Phantasm, curated by Inke Arns, Francis Hunger, and Marie Lechner. The essay accompanies an artwork of the same name, “TODAY’S SELFIE IS TOMORROW’S BIOEMTRIC PROFILE”, on view at the exhibition.
https://www.aclu.org/news/privacy-technology/three-key-problems-with-the-governments-use-of-a-flawed-facial-recognition-service ↩︎
https://www.theatlantic.com/ideas/archive/2022/01/irs-should-stop-using-facial-recognition/621386/ ↩︎
https://www.cbsnews.com/news/irs-lawmakers-urge-drop-selfie-software-id-me/ ↩︎
https://www.irs.gov/newsroom/irs-announces-transition-away-from-use-of-third-party-verification-involving-facial-recognition ↩︎
https://www.nytimes.com/2021/11/23/technology/clearview-ai-facial-recognition-accuracy.html ↩︎
https://www.nytimes.com/2018/02/09/technology/facial-recognition-race-artificial-intelligence.html ↩︎
https://www.aclu.org/blog/privacy-technology/surveillance-technologies/amazons-face-recognition-falsely-matched-28 ↩︎
“In the Age of AI,” Frontline, podcast, November 14, 2019, https://podcasts.apple.com/de/podcast/frontlinefilm-audio-track-pbs/id336934080?l=en&i=1000456779283, accessed December 6, 2021. ↩︎
https://www.classaction.org/news/class-action-accuses-ibm-of-flagrant-violations-of-illinois-biometric-privacy-law-to-develop-facial-recognition-tech ↩︎
https://www.nbcnews.com/tech/security/millions-people-uploaded-photos-ever-app-then-company-used-them-n1003371 ↩︎
“Selfie-Presentation in Everyday Life: A Large-Scale Characterization of Selfie Contexts on Instagram” ↩︎ ↩︎
https://www.gsb.stanford.edu/faculty-research/publications/deep-neural-networks-are-more-accurate-humans-detecting-sexual ↩︎ ↩︎