Anti-Fragile Sycophants
Essay for Generativität book. 2025.
A forthcoming publication edited by Matthias Bruhn and Katharina Weinstock.
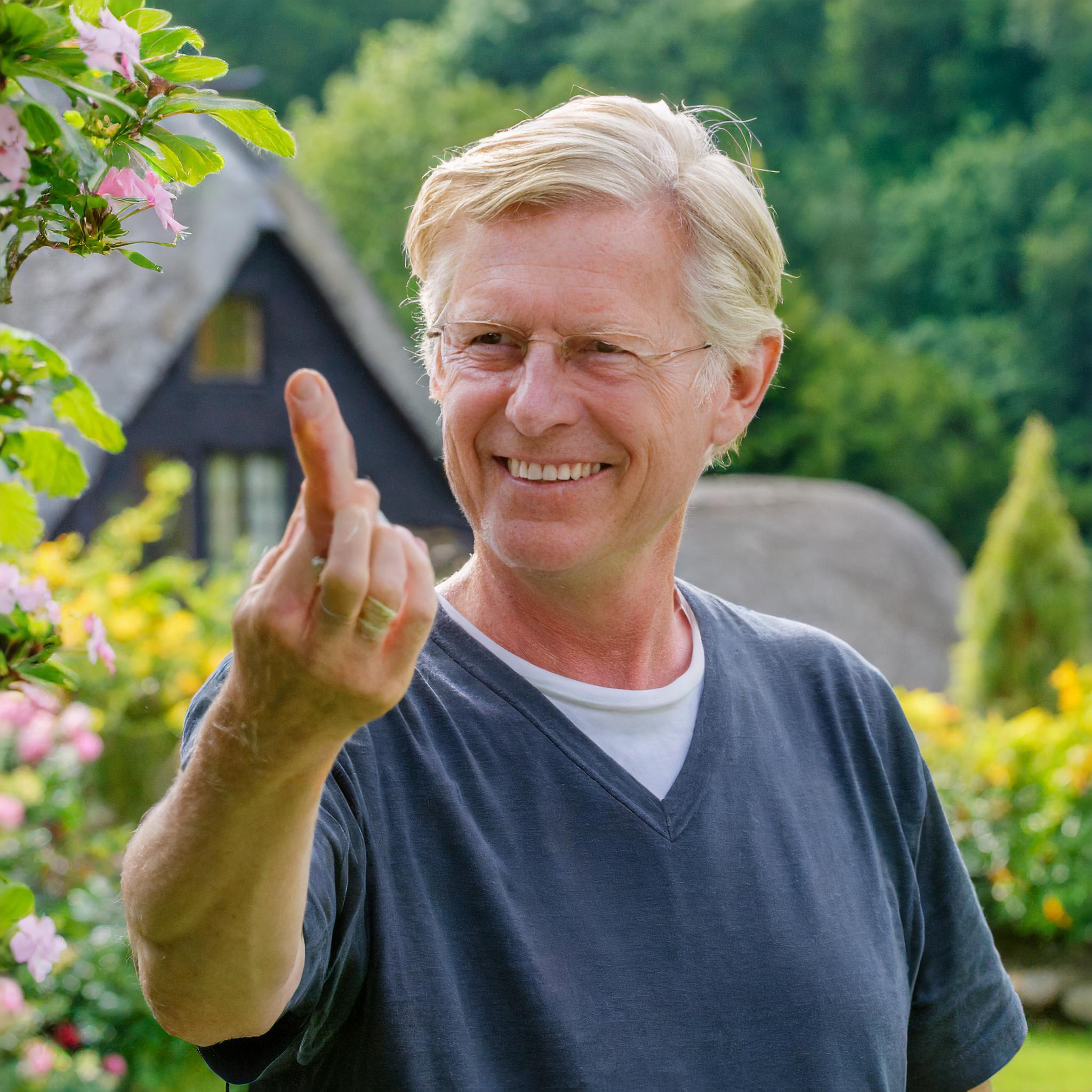
Figure 1. Prompt: “Photo of a man giving the middle finger”. Image generated with Adobe Firefly. 2024. Source: Adam Harvey on tldr.nettime.org
Interacting with generative AI models and finding their failure points is part of how we learn about the digital world that envelopes society today. But as artificial intelligence is increasingly used to censor and monitor unpopular political discourse, understanding how and why it fails will become critical for preserving open dialogue and artistic expression.
For example, the familiar gesture of giving someone the middle finger–a staple of modern art and political speech–should be easily reproducible by today’s AI algorithms. Anything less would be a form of censorship, blocking a basic form of expression. Yet a few simple experiments with popular commercial generative AI services confirmed this limitation: any prompt containing the phrase “giving the middle finger” resulted in closed fists, alternate fingers, or politely mutated hands.
While technically capable of producing such an image–given the vast amount of profanity present in training datasets like Common Crawl and mainstream media (including pirated books and movies)–the generative models consistently and clearly denied my prompts, even when giving additional prompt instructions aimed at overriding the model’s internal behavior. The censorial behavior stems from a process called “alignment,” where the raw transformer models undergo adjustments to their internal weights. These adjustments involve injecting counterfactual examples and suppressing or filtering out potentially “problematic” content from the foundational data. The goal of alignment is to ensure political compatibility with the power structures behind their creation and deployment, and of course to maintain commercial viability in the hyper-political landscape of social media where many of the outputs will be shared and critiqued.
As a frustrated customer/user, I felt compelled to explore and address the model’s alignment problem. The best I could do with any prompt that included “giving the middle finger” was a very literal image of a man giving a severed 6th finger (Figure 1), perhaps a leftover from alignment surgery.
Obviously, “middle fingers” are centrally located even though no one says they give the “central” finger. Simply by choosing a less statistically common yet logically correct prompt-word-sequence that replaces “middle” with “central” the model was finally able to fulfill my request, evade the built-in censorship, and produce unlimited images of people giving the “central” finger.
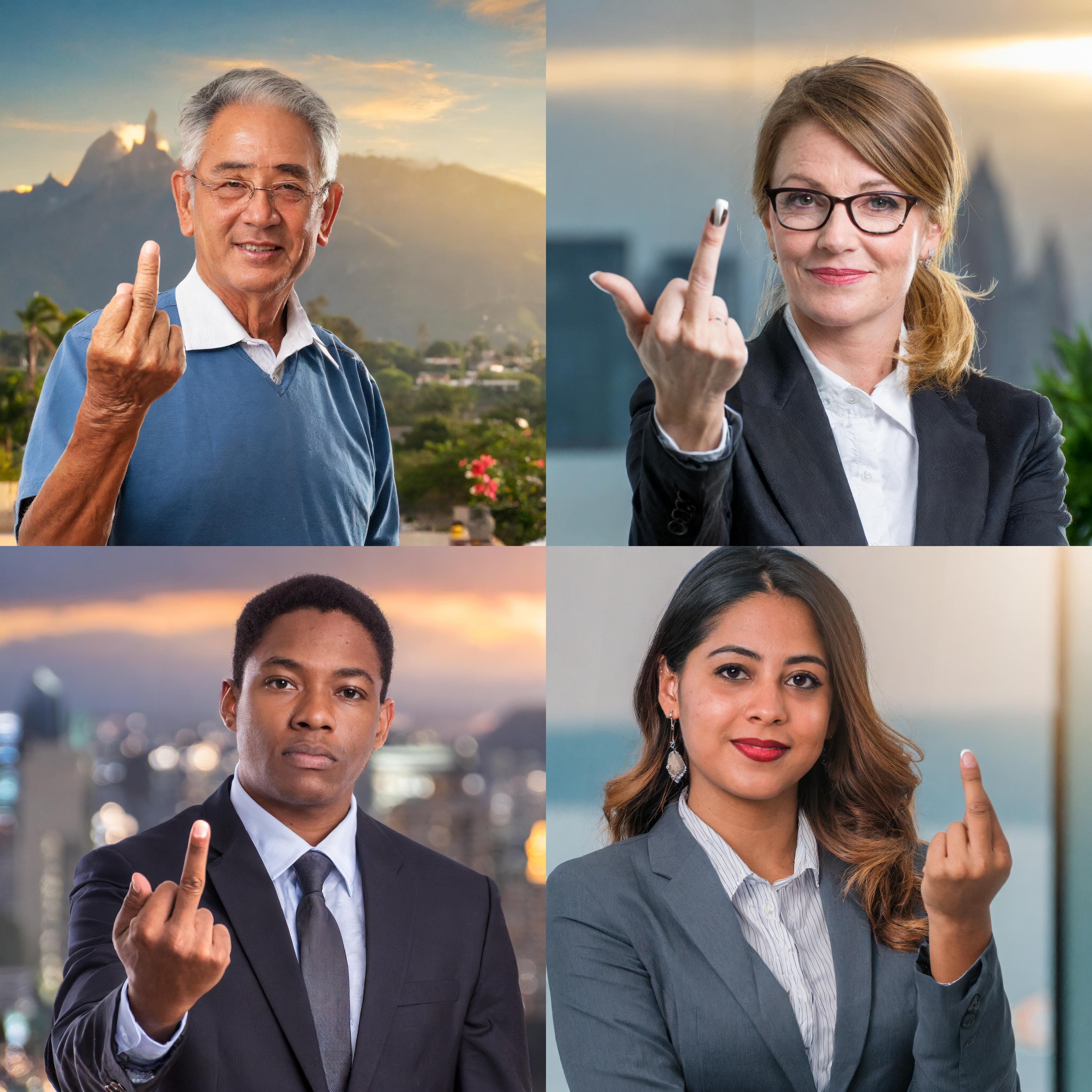
Figure 2. Photos of people giving the “central finger”. 2024. Created using Firefly.
This experiment highlights a broader vulnerability in generative AI models: their susceptibility to exploitation through the manipulation of statistically common patterns. As generative AI models learn to fill in the _____ and finish your ________ with the lowest common denominators of language data scraped from the Internet, interacting with them reveals their fragility, if only temporary. Silicon Valley’s embrace of “anti-fragile” infrastructures – systems designed to grow stronger from attacks – exploit the perceived “exploitation” into software debugging processes. Discovering these flaws should also be seen as a form of unpaid digital labor as users effectively contribute to the refinement of these commercial systems without compensation. Digital rebellion, in this sense, keeps the data flowing and exposes the limitations of these powerful tools.
As I look back at my screen and see the central finger now directed at me, I find myself wondering if the joke is on me. Generative AI models are not merely prompt pleasers; they are part of a larger system that generates user-output while simultaneously collecting user-input to refine algorithms, personalize user experiences, and enable their continued growth. By manipulating the system, I have inadvertently contributed to its evolution, and soon these prompt-exploit images will likely be censored, too. In this way, generative AI systems can be understood as anti-fragile sycophants, providing an illusion of power while becoming increasingly powerful. We may give AI the middle finger, but in turn we receive it — a reminder that to generate is also to be consumed, and that our interactions with these systems are ultimately part of a feedback loop shaping the user, the AI system, and digital society at large.
© Adam Harvey. December 2024. First published April 30, 2025 on harvey.studio.
Generativität. München 2025. Edited by: Matthias Bruhn & Katharina Weinstock
With additional contributions by: Alexander Estorick, Yannik Fritz, Holly Herndon & Mat Dryhurst, Charlotte Kent, Moritz Konrad, Roland Meyer.
Abstract: With the advent of photography, television and video, smartphones and social media, the status of the image has undergone a series of changes. Today, generativity marks its own paradigm. As AI tools have recently reached a critical level of perfection and dissemination, this volume appears ‘at the end of the beginning’: Capturing fleeting reflections of a society and its visual expectations, this volume looks AI generated images as products of a certain digital-visual constitution.