3D Printed Training Data
Essay for Synthetic Cognition by Onassis Foundation, edited by Ilan Manouach and Anna Engelhardt. Published 2022.
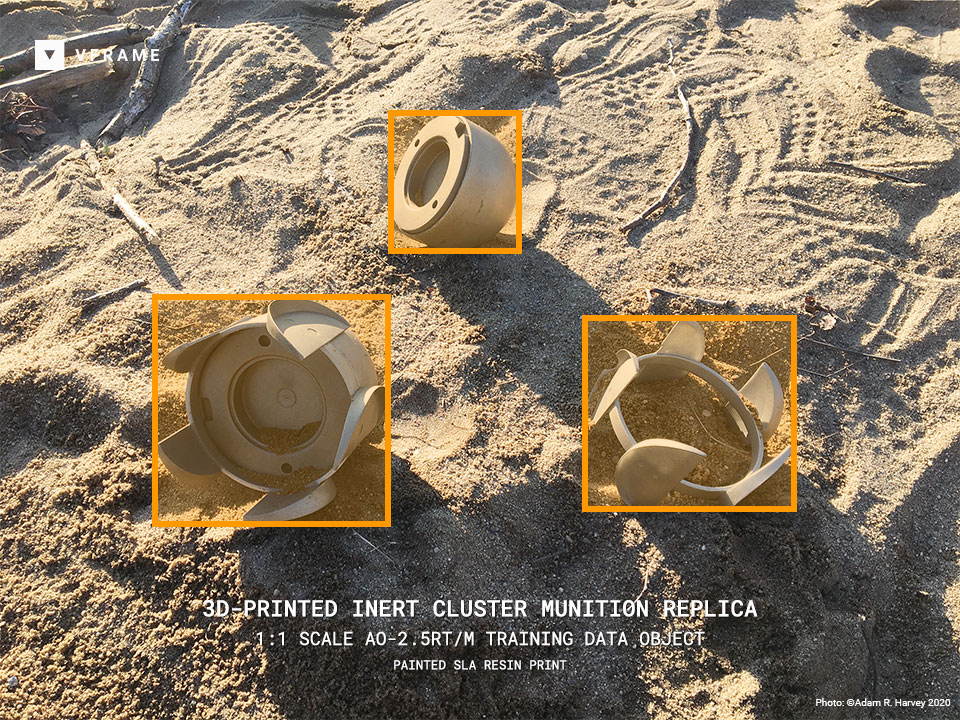
Book available through Onassis at https://www.onassis.org/culture/publications/chimeras-inventory-of-synthetic-cognition/
High-quality training data is an essential component in artificial intelligence systems. But often this data is either over-represented or underrepresented, resulting in biased and incompetent algorithms. As an alternative to the limitations and problems of existing data sources, artists can create their own realities by creating their own data. Using 3D printing, or additive manufacturing, is one new strategy that could contribute to a future where artificial intelligence better aligns with humanitarian applications.
In this example, a cluster munition used in the Syrian conflict has been reconstructed as a 3D model by combining information from publicly available sources including military guides and video documentation. The 3D model is printed, painted, and photographed thousands of times from different angles with different lighting conditions in staged environments that simulate Syrian landscapes. Once these photos are annotated with an associated object-label (i.e. “AO-2.5RT”), they become a source of “3D printed” training data for computer vision algorithms.
The training data is fake but not untrue. To a neural network, there is no objective reality except for the subjective ground truth provided by a developer, which could be either real or non-real. As long as the synthetic data is realistic enough to match the visual features in actual conflict zone videos, it can provide a sufficiently real forgery of visual reality to a convolutional neural network. Fake becomes real when you’re already knee-deep in a simulation.
The 3D printed AO-2.5RT cluster munition pictured above was created for VFRAME.io, an open-source computer vision project that works with human rights researchers to help document, verify, and archive footage from conflict zones. By using 3D printed objects as training data, object detection algorithms can be built safely under controlled conditions, without any of the inherent dangers of handling or documenting explosives in active war zones.
Although this 3D-printed data object is highly specific, the concept could be extended to other areas. Computer vision, and artificial intelligence in general, typically analyzes the future through the past. But many researchers have shown that this approach inherits the injustices embedded in history. Indeed, a growing number of computer vision datasets have been deprecated or deactivated because of their problematic, misogynistic, and racist taxonomies. Rather than looking backwards to outdated data, artists can also look forwards by imagining and creating new AI training data sources that yield new ground truths which, in effect, yield new computational logic.
As Geoffrey Hinton, considered by some as a “Godfather of Deep Learning”, points out: “our relationship to computers has changed”. “Instead of programming them, we now show them and they figure it out.”1 If this is true, then perhaps it is no longer only the engineers, but also the artists and designers that will play a major role in guiding the future of AI.
For more information about how 3D printed training data is being applied to computer vision for humanitarian applications visit VFRAME.io
Text: © Adam Harvey 2021. All Rights Reserved.
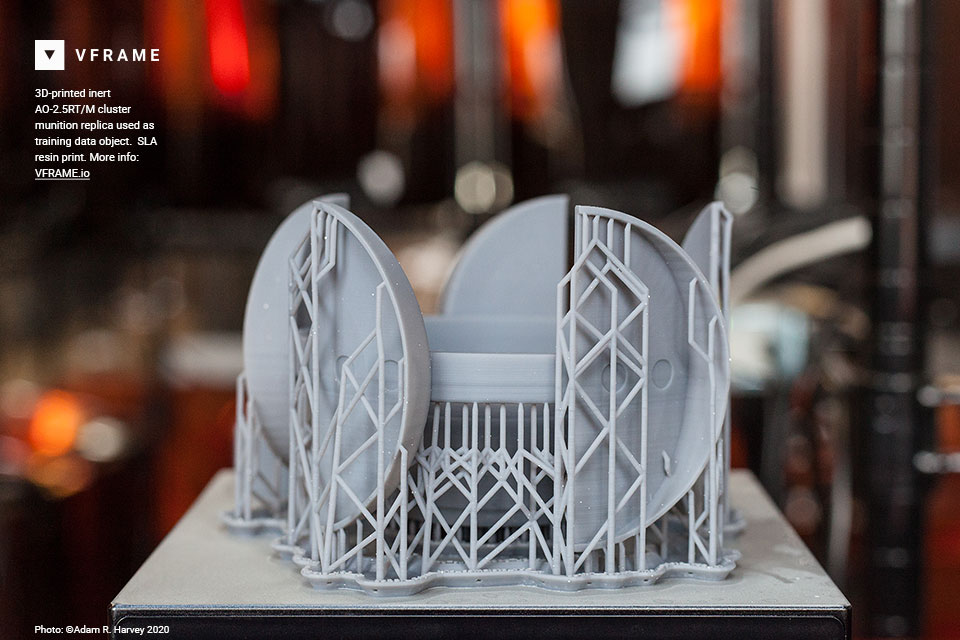
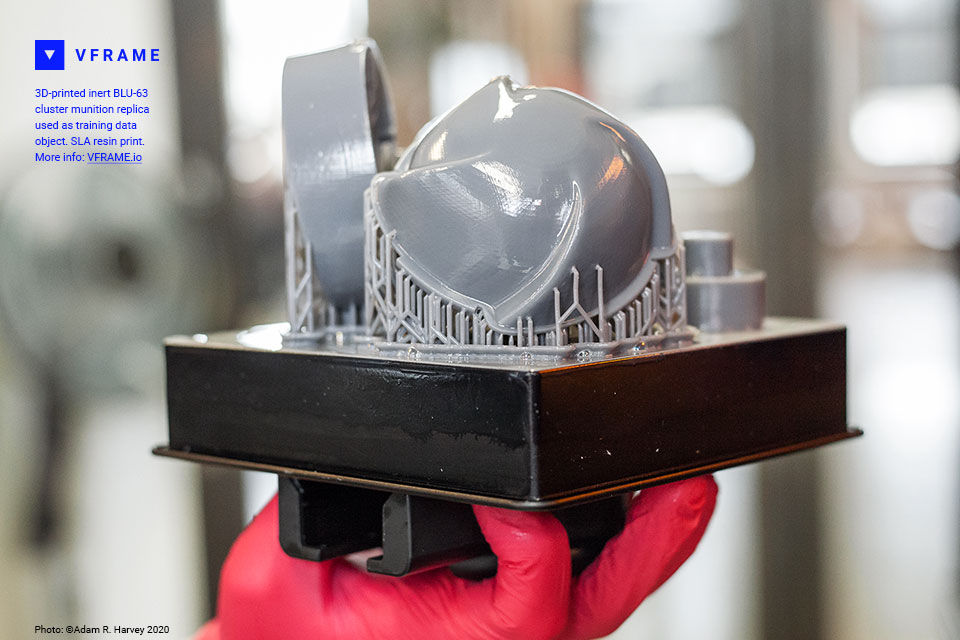
3D printed fins for the AO-2.5RT cluster munition.
“Heroes of Deep Learning”. Aug 8, 2017. YouTube.com.https://www.youtube.com/watch?v=-eyhCTvrEtE ↩︎